A Q&A with Steve Chadwick, Intel Principal Engineer of Manufacturing IT
The Fourth Industrial Revolution is upon as. A wide variety of manufacturers are embracing
advanced technologies running the gamut from the internet of things and connectivity to data
analytics and automation in an effort to create new levels of operational efficiency and business
value. To gain a better understanding of what this process looks like from a manufacturer’s
perspective, Enterprise IoT Insights discussed Intel’s approach with Principal Engineer of
Manufacturing IT Steve Chadwick.
Q: How has your company approached the process of digitally transforming its manufacturing
operations?
A: For Intel IT, digital refers to the use of technology that generates, stores and processes data.
Transformation refers to a fundamental change to our organization’s day-to-day business – from
the types of products and services we produce to how we deliver them.
For manufacturing, automation and the effective use of data are central elements of Intel’s
competitive strategy. We have been actively engaged in leveraging technology advancements to
improve our manufacturing process. While we’ve used automation in Intel’s factories for several
decades, we continue to improve it through deployment of widespread IoT and predictive analytics solutions, which decrease time-to-market, improve resource utilization, increase yield
and reduce costs.
Q: How have connectivity and the internet of things changed the way Intel runs its business?
A: Intel’s manufacturing process runs 24 hours a day, 7 days a week, 365 days a year. Running a
factory non-stop requires considerable investment in our equipment. By utilizing the sensors in
the tools, we are able to maximize the equipment availability and utilization. We have been able
to create a data visualization tool that presents factory engineers with graphs that distinguish
critical errors from noncritical errors and show where manufacturing equipment is running well
and where opportunities exist to improve efficiency. This focuses attention on the most
meaningful improvements to increase equipment availability and yield.
Our goal is to consume this data and turn it into insights. Leveraging these sensors is not new,
but the data volume that is generated has been rapidly growing. For example, we have used
advanced analytics to process more than five billion data points per day per factory, which
enables engineers to extract needed information in 30 seconds instead of four hours. Now
engineers can focus on solving problems and designing solutions instead of extracting data.
What is new, is the ability to utilize our IoT technology to drop a sensor anywhere it’s needed.
This makes monitoring locations that were previously difficult to reach, within grasp. This alone
has opened additional vectors of data that can be turned into information previously
un-accessible.
Q: What were some of the problems you needed to solve?
A: Sensors running non-stop have the ability to generate huge volumes of data. The ability to
collect this data is not trivial. As stated previously, one Intel factory produces over 5 billion data
points per day that are collected, and we have several of these large factories. The ability to
collect all of the data, store it and then turn it into meaningful information requires a lot of
thought.
For example, if there are 5 billion points of data per day, how is that data stored in a way that
insights could be delivered in a timely fashion? How is a trend presented to an engineer that
allows them to understand their manufacturing tools better? How do you trend days of data?
These are all simple questions but given the large volumes of data, the underlying structure has
to scale.
Q: Can you take me through the technologies used to solve those problems?
A: It’s more of a process than a specific technology. When tackling a problem, I think it’s useful
to consider the following model.
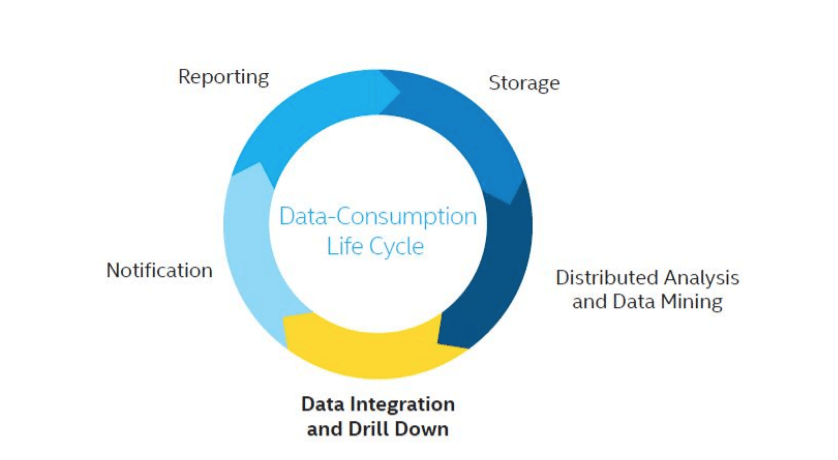
This is what I call the data life cycle. This model outlines the types of questions that we ask
about the problem and helps guide us to the solution. Here is a quick explanation.
- Storage – This is the first step in our model. What are the storage requirements? How fast does the data need to be written and retrieved? What volume of data is required to make decisions and how long should we keep it?
- Distributed Analysis and Data Mining – This step is often computationally expensive as well as input/output [IO] intensive. The systems have to consider items such as network bandwidth, distributed computing and potentially server farms. In this step, the correlations between variables are discovered.
- Data Integration and Drill Down – Sometimes data exists in silos. The real value is when datasets are brought together. This data integration provides the ability to move seamlessly across the datasets and drill down into specific items, which becomes increasingly important, as well as difficult.
- Notification – If a signal is discovered or if there is information to be communicated, how will that communication occur? When is a Short Message Service [SMS] appropriate vs. leveraging another automated system? Machine-to-machine communication is more efficient but sometimes there are other factors to be considered.
- Reporting – Generating a report that is actionable should be the goal. The team shouldask itself what information the end user needs to see to make an informed decision.
When the team works through the data life cycle, this process will help identify both the
opportunities as well as potential sticking points. This also provides a framework to help scope
the different areas of work and to bring in additional resources with expertise to help when
needed.
Q: Separate from the technology, were there any implications to your workforce related to digital
transformation?
A: Intel IT spent USD $1.4 million in 2016 on training IT employees. By tracking emerging and
declining industry skills and investing accordingly, we create an agile, positive-thinking
innovative workforce that embraces our collaborative One IT culture. We encourage employees
to take a range of courses because our most valuable employees have both business acumen and experience across disciplines. We also build management depth through leadership development
and succession planning. Management training and networking nurtures effective leadership,
which in turn powers business transformation.
Q: What are some of the key lessons you learned from the process?
A: In general, the approach that has worked well is to start small. One of the concepts in lean
manufacturing is the idea of small incremental improvements. This approach has worked well in
the past. The idea is that by starting with something simple and quick there is an opportunity to
engage with the end-user. This working model can then develop over time. As it develops, the
complexity will typically increase, but it grows in manners that maybe were not initially
apparent.
Q: What, if any, problems did you encounter through the implementation?
A: With any large project, there will be issues. Is the data generation outpacing the storage
available? Are we collecting the best data possible? How long should we retain this data?
These are common questions that will arise during a project.
In order to address these issues, it’s often advantageous to come back to the initial problem
statement. What problem am I solving and what is the value of solving that problem? This
simple revisiting of the objective often helps re-align the team. Past this, having a structured
approach to solving problems is invaluable. Combining this approach with the idea of trying to
keep the approach as simple as possible often leads to over-coming the typical project hurdles.
Q: What guidance would you offer your colleagues looking to transform their manufacturing
operations?
A: There are three items that are important to consider when starting a project. First, what
problem is being solved? If the team is new, start with a simple problem. The problem does not
have to have the highest value to the company, but it should have value. Consider this a way for
the team to form and learn. Once a few projects are achieved with success the team will gain
confidence and grow into solving larger projects with higher value and they will have the
necessary experience to achieve a positive outcome.
Second, work through the data life-cycle (as outlined above). Each section likely has different
stakeholders – which may require a few different meetings. However, by outlining the steps, the
team will start to form some ideas on the technologies to use along with gaining a better
understanding of the customer’s requirements.
Third, when it comes to implementation, keep the design as simple as possible. Perfection is
often not required out of the gate. Work closely with your end customer to solve that initial
business problem. Can 80% of the problem be achieved without a lot of complexity? This is
what should be pursued first. This approach allows the team to learn and provide incremental value. As the team approaches the 80% milestone there will be considerably more depth to
tackle the remaining portion of the project, which oftentimes is more complex.
For additional information on this topic, check out the following resources provided by Intel.
- Intel IT Annual Performance Report
- Automated Manufacturing 101
- Putting Sensors to Work in the Factory Environment: Data to Information to Wisdom
- Inside IT: Improving Manufacturing with Advanced Data Analytics
- Joining IoT with Advanced Data Analytics to Improve Manufacturing Results
- Using Big Data in Manufacturing at Intel’s Smart Factories
- Integrating IoT Sensor Technology into the Enterprise
To hear more from Steve Chadwick and other industry leaders, join Enterprise IoT Insights in
Austin, Texas, for the Smart Manufacturing Forum, set for April 17-18.