There is a dizzying array of data available to telecom operators on the status, performance and health of their networks; at various speeds and levels of granularity; focused on end-user-experience, peak network capability, or dozens of other potential indicators.
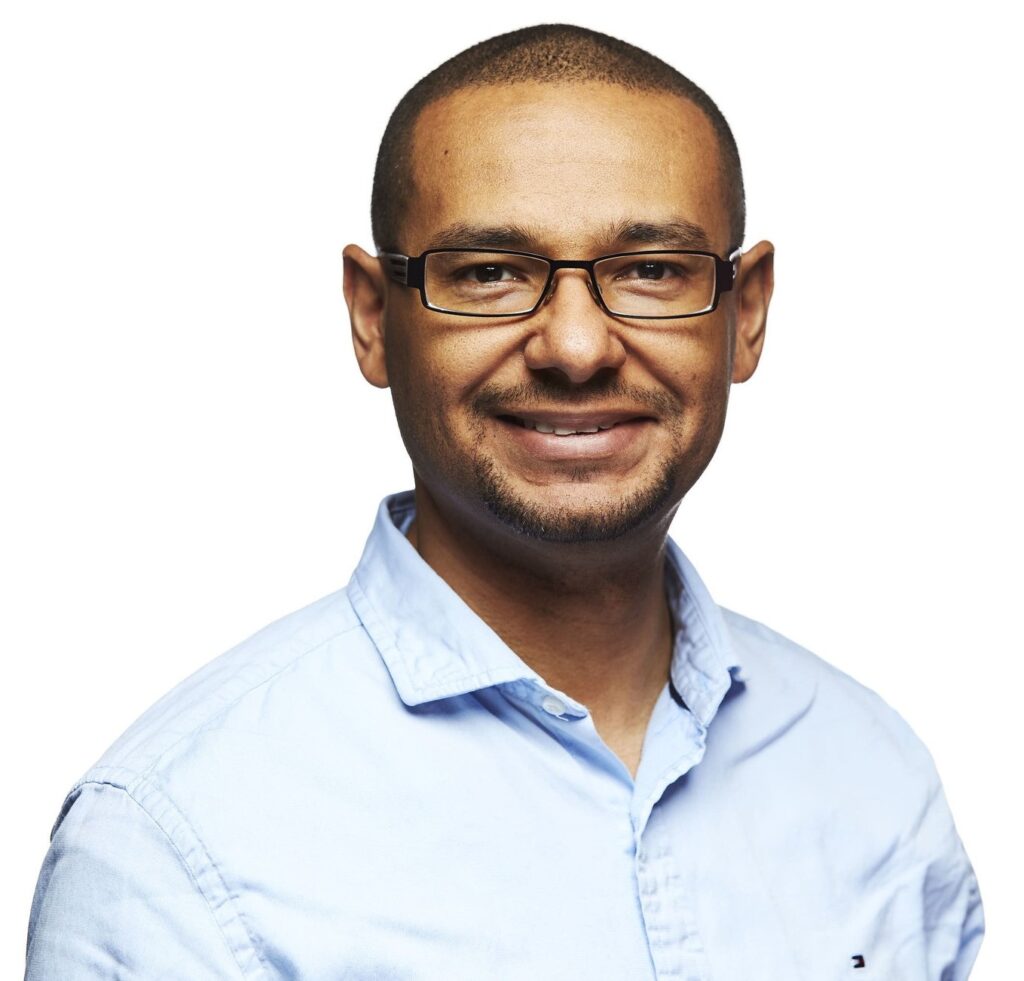
Operators face considerable challenges in making the best use of that data and translating it into actionable intelligence to validate, assure and optimize network operations. RCR Wireless News reached out to Ayodele Damola, director of artificial intelligence/machine learning strategy at Ericsson, for his perspective on those challenges and the current landscape and trends in gathering, analyzing and leveraging network data as well as the use of AI and ML in telecom networks.
This Q&A was conducted via email and has been lightly edited.
RCR: As the industry moves further into 5G and 5G Standalone deployments, as well as disaggregation and cloud-native networks, how would you describe the challenges surrounding navigating network-related data? Is it a matter of new sources (i.e., microservices leading to more data granularity), new volume or scale of data, or the velocity/speed at which data is available — or some other factor?
The main challenge navigating network data is the increase in complexity. As 5G gains traction across the globe, communication service provider (CSP) networks are becoming even more complex. The complexity is due to the new set of services being offered, the increase in number and types of devices in the network, availability of new spectrum frequencies and bands, and the evolution of physical networks to virtualized networks. This complexity equates to an increase in the volume of network data generated; network data generated in different time frames in addition to the presence of more variety of network data. If we take, for example, the reference parameter count evolution in 3GPP radio access networks (RAN) – we saw that with 2G networks when we had just a few hundred reference parameters that needed to be configured. In 5G, that number has grown to several thousand parameters. In 6G, we anticipate an even larger number of reference parameters. Increase in complexity is also reflected in ‘overabundance of data’ – it becomes difficult to find relevant data without innovative filtering and aggregation. Another issue is the lack of standardization of data across proprietary vendor equipment leading to difficulties in leveraging the data for insights. Yet another issue is data management – today, it is an afterthought consisting of brittle system information (SI) driven pipelines which are inefficient. Basically, data is duplicated and hard to govern. All these factors put new demands on the level of effort needed to manage and control the network, and thereby lead to an increased CSP operation expense (OpEx) and eventually increased capital expense (CapEx). It is becoming clear that it will no longer be possible to manage networks in a legacy way where network engineers look at dashboards and make changes manually to the network. This is where a technology like AI enters the picture, a technology that enables automation and thereby reduces complexity.
RCR: Do you think that telecom operators, by and large, have a good handle on their network data? What do you think they do well, and where is there room for improvement?
Access to network data is an important component of most CSP strategies today. Network data from multi-vendor networks has been somewhat of a challenge for CSPs because each vendor has slightly different network node interfaces – hence, the format and syntax of the data may be different across different vendors. Often, CSPs lack a consistent strategy for data persistence and exposure. Different storage mechanisms (e.g., data lakes) and retention policies complicate the scalable handling of data volumes. In the future, open standards promise to alleviate this challenge, specifically by the introduction of the Service Management and Orchestration (SMO) framework defined by the ORAN Alliance, which provides a set of well-defined interfaces enabling CSPs to access and act on data from both purpose-built and virtualized multi-vendor and multi-technology networks. Network data is exposed via open interfaces (e.g., R1, A1, O1, O2 etc.) to the different SMO functions.
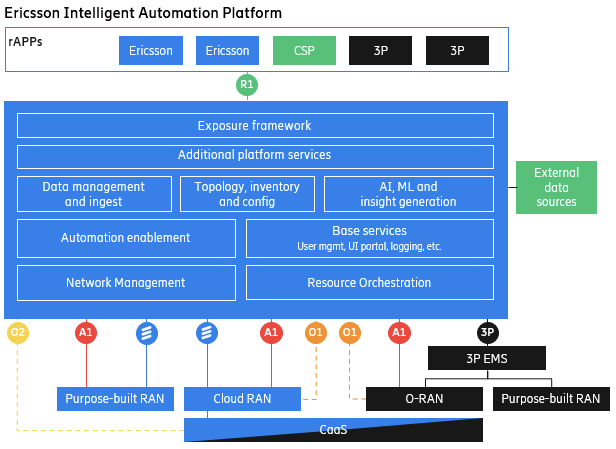
Within the SMO (our implementation is called the Ericsson Intelligent Automation Platform) the Data management and ingest function enables CSPs to efficiently and securely ingest and manage data. The AI/ML and insight generation function enables the ability to process and analyze the data, deriving insights and facilitating actuations.
RCR: What do network operators want to use their data for? Can you give the top three uses for network-related data that are the primary interest of MNOs?
Market research earlier commissioned by Ericsson shows that CSPs leverage network data across many use cases, with the top three being:
- Customer Experience Management: Solution helps CSPs predict customer satisfaction, detect experience issues, understand root causes, and automatically takes the next best action to improve experience and operational efficiency leading to churn reduction and increased customer adoption.
- Security/Fraud & Revenue Assurance: Addresses security management and revenue assurance including billing and charging.
- Cloud & IT Operations: Automation of cloud and IT management operations including administrative processes with support for hardware and software, and the rapid isolation of faults.
Additionally, other important use cases include Network Management & Operations, Enterprise Operations, Service Assurance for RAN & Core, Network Design and Optimization, RAN Spectrum and Traffic Management.
RCR: What role is AI/ML playing in networks at this moment in time? People are very interested in the potential — what is the current reality of practical AI/ML use in telecom networks, and could you give some real-world examples?
AI/ML promises huge potential when it comes to optimizing and automating CSP networks. The industry is still in its early days, but some problems that have been solved with AI/ML show substantial gains. The full systemization of CSP networks based on AI/ML, also called AI-native networks, is still some years away. When it comes to the RAN specifically, Ericsson believes that AI/ML will play a key role in the following areas:
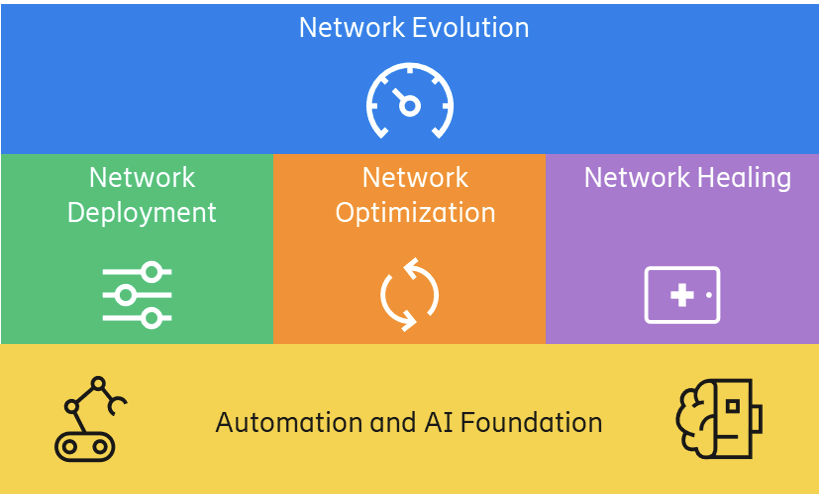
- Network evolution: Enhances network planning with more efficient RF planning, site selection and capacity management. Improves network and service performance and enables new revenues by data driven and intent-based insights and recommendations.
- Network deployment: Handles provisioning and life cycle management of complex networks with optimal costs and speed to market.
- Network optimization: Intelligent autonomous functions to optimize customer experience and return on investments, e.g., RF shaping, traffic and mobility management, energy efficiency etc.
- Network healing: Service continuity and resolution of both basic and complex incidents, delivering high availability while keeping the operation costs at a minimum.
- AI and automation foundation: Enables faster TTM for – and trust in – high performance AI and automation use cases by means of openness and flexibility.
In addition, Ericsson believes CSPs will benefit from an end-to-end managed services operations solution enabling the intelligent management of CSP networks and services to provide superior connectivity and user experience. Powered by advanced analytics and machine learning algorithms, CSPs will benefit from the ability to predict potential network issues caused by hardware, software, or external factors such as weather disturbances or customer behavior patterns.
Practical AI/ML use in telecom networks today:
- Capacity Planning: Provides the ability to perform proactive planning based on traffic forecast in combination with AI/ML prediction of utilization KPIs. The outcome will be the optimum capacity Expenditure to meet a certain QoS level. Forecast predictions, bottleneck identification and network dimensioning are the main use cases. Benefits include 20-40% CapEx savings less carrier expansions compared to traditional approach, and 83% increased operational efficiency increased operational efficiency on dimensioning tasks.
- Performance Diagnostics: A solution that analyzes CSPs’ RAN to detect and classify cell issues. Identified issues are further investigated down to root cause level, enabling fast and accurate optimization of end-user performance. Benefits include: 30% increase in capacity per optimization full time employee (FTE), and 15% better downlink speed in cells with issues.
- Improved spectrum efficiency: By collecting adjacent cell data in real time, it is possible to optimize radio link performance using pattern recognition. Benefits include: 15% improved spectrum efficiency and 50% increased cell edge DL throughput.
- Sustainability: An autonomous mechanism using AI/ML technologies and closed-loop automation to reduce daily radio network energy consumption by up to 25% with zero impact on user experience.
When it comes to the potential of AI/ML in CSP networks, we envision a journey with several steps:
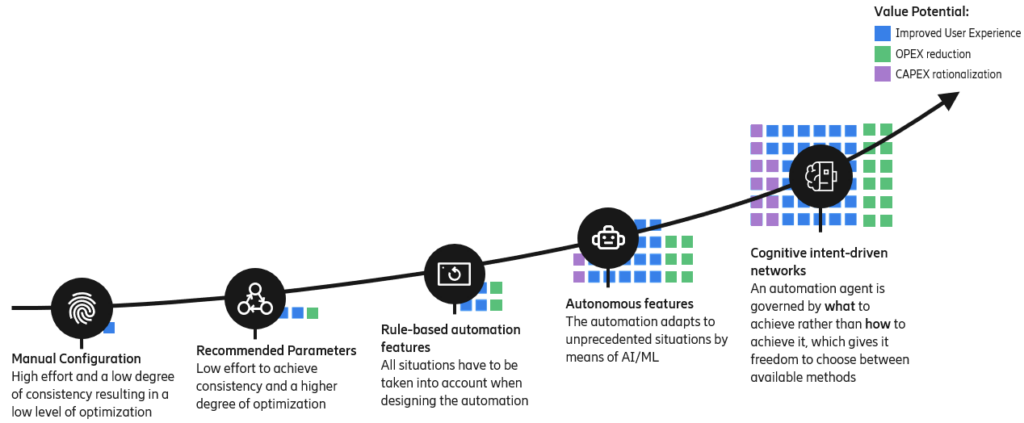
In the earliest step, there was complete human intervention as the network was manually configured. In the next step, the network configuration was still performed by humans, but the effort level was reduced to the configuration of a recommended set of parameters. In the rule-based step, the human role was to create a set of rules which then controlled the network; this required that the human developers have a deep understanding of how the network functions. We are currently transitioning to the step with autonomous features where we have AI/ML models adapting to new situations and thereby giving CPS more automated control. Going forward, the vision is that the network will evolve to fully autonomous with no human intervention other than setting intents by which the network operates – basically, providing the ‘what’ requirements to the network with the network performing the needed ‘how’ actuation and control. It is a journey similar to the evolution of cars from manually controlled to fully self-driving.
RCR: In terms of edge vs. centralized cloud, is most data processing still centralized or do you see things actually becoming more distributed and happening at the edge? Is it different for telco workloads vs. enterprise workloads?
It is important to distinguish between data collection, typically for the purpose of training an AI/ML model, and inference, which entails acting on new data by a trained model. The process of collecting data will entail cleaning and sorting the data and then using this data to train models. While the data collection will happen out in the network in a distributed manner, the data processing will typically be done centralized. After the model is trained, the deployment of the model in the network could be either distributed or centralized, depending on the use case. Given the large volume of network data and given its real-time nature, inferencing of telco workloads will typically be more distributed compared to enterprise workloads.
RCR: There was for some years a big push toward “data lakes” and storing as much data as possible to sift through for business intelligence. Is this the case for network data as well, or is there more preference for real-time intelligence? What does “real time” actually mean right now, how close can it get?
The preference for real time intelligence will depend on the use case. Network data is generated across the network in different time frames, and we can classify use cases based on the network data into fast-loop use cases (microsecond timeframe) and slow-loop use cases (timeframe of days or even weeks) and all the in-between. The nature of the use cases across different timeframes will differ significantly. A real time or fast-loop use case is, for example, radio scheduling made in microseconds executed locally within a network node and typically fully automated i.e., done without a human in the loop. Slow-loop use cases will be focused more on long term network trends like network benchmarking, requiring network wide coordination with more time available to make a decision and will likely entail interaction with a human. Data lakes are then suited for the two upper loops in the picture below, while the time sensitivity of both the data and the decisions made in the two lower loops speak against persistence of the decision-bearing data in any type of data lake.
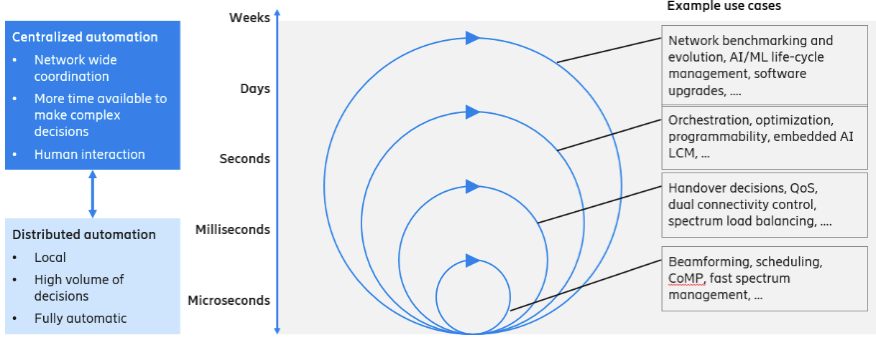
RCR: What data needs, challenges or changes should telecom operators be planning for now that you see coming in the next 3-5 years?
I see three things:
- Quality data: CSPs are challenged with how to define and develop a quality data approach from which all AI solutions can be delivered. The issue is that data is still contained in silos across most CSPs, from legacy systems to new systems. AI/ML will only make a difference if clean data is available from all sources. Hence, investing the time in and then training of AI algorithms with the right data will be essential to reducing false alarms and in improving AI effectiveness. An adjacent problem is the issue of relevant data – some of the data generated has very little to no entropy (i.e., its usefulness is limited) and identifying such data is an ongoing challenge.
- Data platform: All CSPs require a robust platform to aggregate, sanitize, and analyze the data. While there are several potential solutions in the market, there are concerns of privacy, security, and vendor lock-in that make data platform selection difficult.
- Data strategy: Many CSPs lack an end-to-end data strategy which covers data governance, simplification and automated collection, and data analysis. While some CSPs have a Chief Data Officer (CDO), many CSPs have not yet been able to enforce a companywide data strategy across various organizations leading to disconnected islands of data.