Scan the tech headlines, and you can’t escape the impression that artificial intelligence (AI) is about to transform practically every industry. Usually though, the picture these stories paint of AI-driven disruption is still hypothetical. For the organizations running the world’s biggest data centers, however, the transformation has already begun.
The extraordinary performance demands of generative AI (GenAI) applications, and their explosive growth, are pushing current data center networks to the limit. In response, hyperscalers and cloud service providers are scrambling to add thousands of graphics processing units and other hardware accelerators (xPUs) to scale up AI computing clusters. Connecting them will require networks that deliver high throughput and low latency at unprecedented scales, while supporting more complex traffic patterns, such as micro-bursts. Data center operators can’t meet these needs by simply adding more racks and fiber plants as they have in the past. Ready or not, they have no choice but to reimagine data center architectures.
What will emerging AI-optimized data center fabrics look like? Which interface technologies will they use, and how will they affect market uptake of 800-Gbps transport and next-generation Ethernet? Data center operators are working through these questions now, but even in these early days, we’re starting to get some answers.
Meeting the AI networking challenge
Dell’Oro Group estimates that AI application traffic is growing by a factor of 10x, and AI cluster size by 4x, every two years. Part of this growth stems from increasing adoption of AI applications, but much of it derives from the growing complexity of AI models themselves, and the extraordinary scale of xPU-to-xPU communication that comes with them.
To understand why, it helps to review what AI workloads actually entail. There are basically two phases of workload processing that AI clusters must support:
- Model training involves ingesting massive data sets to train AI algorithms to find patterns or make correlations.
- AI inferencing entails AI models putting their training to work on new data.
Neither phase will have a major short-term effect on front-end access networks, which are primarily used for data ingest. In back-end clusters, however, intensive AI training and inferencing workloads demand a separate, scalable, routable network to interconnect thousands, even tens of thousands of xPUs.
Many cloud service providers are already struggling to achieve terabit networking thresholds for current AI workloads. With AI models growing 1,000x more complex every three years, they can expect to need to support models with trillions of dense parameters in the near future. To meet these demands, data center operators need network fabrics that deliver:
- Extremely high throughput: AI clusters must be able to process extremely compute- and data-intensive workloads, and support thousands of synchronized jobs in parallel. AI inferencing workloads in particular generate 5x more traffic per accelerator and require 5x more bandwidth than front-end networks.
- Extremely low latency: AI workloads must progress through large numbers of nodes, such that excess latency at any point in the system can translate to significant delays. According to Meta, about a third of elapsed time in current AI workload processing is spent waiting on the network. For many real-time AI applications (from advanced computer vision to industrial automation to smarter customer service chatbots), such delays can lead to poor user experiences or even render applications unusable.
- Zero packet loss: Packet loss can be a significant contributor to latency as networks attempt to buffer or retransmit missed packets. This is a big problem for AI model training in particular, where workload operations can’t even complete until all packets are received.
- Massive scalability: To support more advanced AI applications, model training and other distributed workloads must be able to scale efficiently to billions—soon trillions—of parameters across thousands of nodes.
Together, these requirements underscore the need for new scale-out leaf and spine architectures for back-end AI infrastructures. Moderate-sized AI applications using thousands of xPUs will likely require rack-scale clusters with an AI leaf layer. The largest AI clusters connecting tens of thousands of accelerators will need data center-scale architectures with routable fabrics and AI spines.
Evolving interfaces
One of the biggest open questions surrounding AI is how emerging applications will affect adoption of next-generation interface technologies like 800G Ethernet. This too represents an unfolding story, but we’re starting to get some clarity on how the market will shake out.
Dell’Oro Group forecasts that front-end data center networks will largely follow traditional upgrade timelines, with 800G Ethernet making up one third of front-end network ports by 2027. In back-end networks, however, operators are migrating much more quickly. There, 800Gbps interface adoption will grow at triple-digit rates, encompassing nearly all back-end ports by 2027.
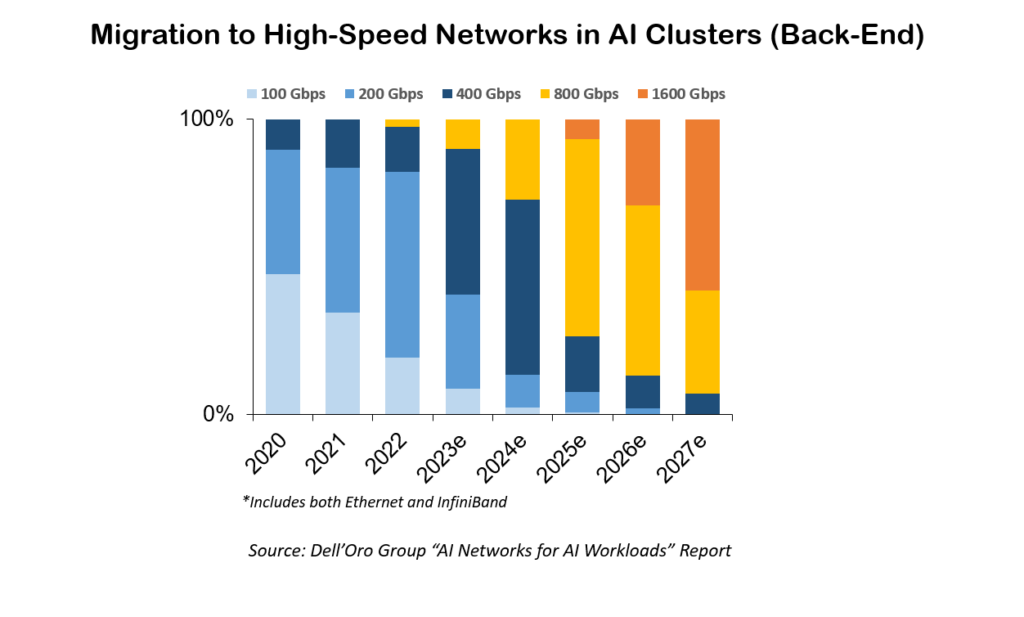
We’re also beginning to get answers on which interface technologies operators will use. Dell’Oro Group expects most operators to continue using Ethernet in front-end networks for the foreseeable future. Back-end networks will be more mixed, as operators weigh the benefits of familiar, cost-effective Ethernet against the lossless transmission capabilities of proprietary InfiniBand. Some operators targeting AI applications that cannot tolerate unpredictable performance will choose InfiniBand. Others will use Ethernet in conjuncture with new protocols like RDMA over Converged Ethernetversion 2 (RoCEv2) as well as per flow congestion control to enable low-latency lossless flows. Still others will use both.
Looking ahead
For now, there is no single answer for the optimal AI cluster size, interface type, or migration path to high-speed interfaces. The right choice for a given operator depends on a variety of factors, including the type of AI applications they plan to target, the bandwidth and latency requirements of those workloads, and the need for lossless transmission. But considerations extend beyond the merely technical. Operators will also need to consider whether they plan to support intensive model training workloads in-house vs. outsourcing them, their preference for standardized vs. proprietary technologies, their comfort with different technology roadmaps and supply chains, and more.
Regardless of how operators answer these questions, one truth is already clear: given how rapidly AI applications are evolving, proper testing and validation is more important than ever. The ability to validate standards compliance, interoperability, and timing and synchronization in particular are essential capabilities for rapid migration to next-generation network interfaces and architectures. Fortunately, testing and emulation tools are evolving alongside AI. No matter what tomorrow’s AI data centers look like, the industry will be ready to support them.