If Jenson Huang says jump, the markets say ‘how high?’. Right? Such is the planet-shifting potential of artificial intelligence (AI), apparently – in capitalist minds today. At least until a Chinese upstart AI-outfit says, ‘wait; not that high’, and enough financial chaos is unleashed to reveal the volatility at the heart of the tech industry’s plan to disrupt the global economy, and remake its systems of power. So, what? China makes it cheaper, and AI is over-hyped?
Well, who knew?
AI cannot be ignored, clearly, and might just do some good, as well as make some people even richer; and NVIDIA boss Huang, in charge of the on/off-richest company on the planet, should not be ignored – especially when he takes to the stage, as he did at CES in early January, to hail ‘agentic AI’, like an empowered version of generative AI that makes decisions, as a “multi-trillion dollar opportunity”. Meaning what, exactly?
For most people in most enterprises – taking second-hand cues off tech savants like NVIDIA – it means more tech terminology to contend with. Agentic AI? Physical AI – he said, too, right? Because most of us – in the trenches – are only just familiar with generative AI. Do these new terms mean we are closer to ‘artificial general intelligence’ (AGI) – so hellishly portrayed in sci-fi fantasies, so lustfully pursued by the tech barons at the front of Trump’s victory parade?
We need to know – in pub terms – what these modish AI concepts mean. So maybe it is time to take stock – before we ask about our own places in all of this.
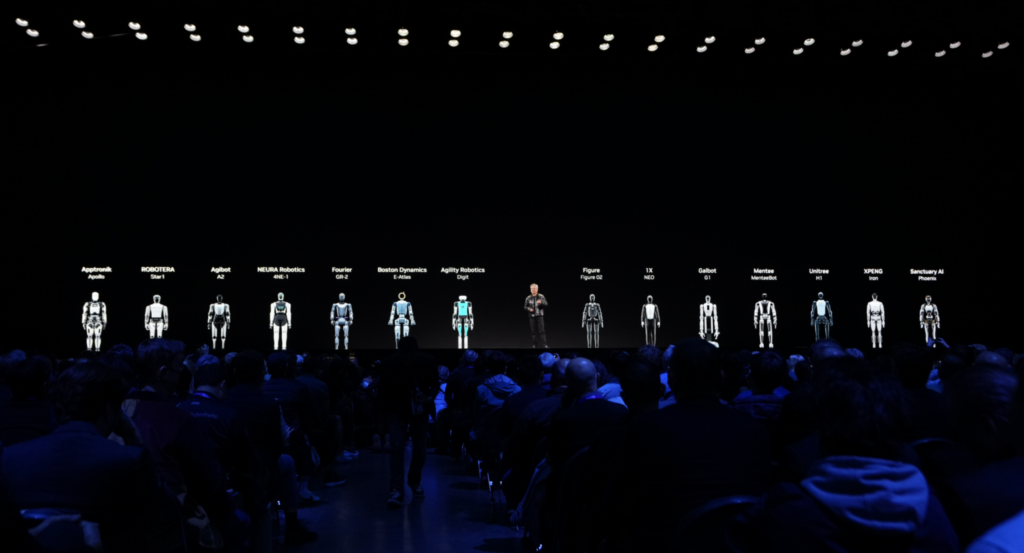
All-conquering AI
All of these terms – generative AI (gen AI), agentic AI, physical AI, and artificial general intelligence (AGI) – relate to different aspects of AI. But they present different concepts, as well, to be leveraged differently by enterprises. In order, their basic functions go from simple content creation (generative AI) to autonomous decision making (agentic AI), to physical/robotic interactions (physical AI) – and ultimately to proper independent thought processing (AGI).
In the end, AGI describes the broad notion to create some kind of sentient intelligence. It is theoretical for now, embodying the sci-fi dream of ‘strong AI’ – even if the tech bros talk like it is around the corner. But these other AI concepts, classified as ‘weak’ (or ‘narrow’) AI, are very real, suddenly – enough for stock markets to bounce on trade-show keynotes and leftfield commercial innovations. They describe specialised systems for specialised tasks.
Unlike quasi-conscious AGI, they do not generalise beyond their pre-schooled functions. They promise to streamline siloed operations – and even, chugging on the Kool-Aid, to hyper-charge them to create highly-directed production capacity, and proper industrial innovation. Which is the marketing logic with every Industry 4.0 technology, of course – except the AI hype is all-consuming because AI wants to automate brain systems, rather than just physical ones.
In other words, it is not just about replacing human functions in enterprise systems but also imposing human-like logic, creativity, and control onto them – whether in isolated industrial disciplines, or in the whole global economy. And everywhere else, in all of society – if riotous capital AGI development goes uninterrupted and unchallenged. In theory, ‘weak’ AI keeps middle-managers in jobs; but, bottom line, AI will replace white- and blue-collar workers alike.
And really, apart from a handful of zealots at the very top of the AI money tree (the 26 richest billionaires own as much as 3.8 billion of the world’s poorest people; or half the global population), everyone’s livelihood is at stake. Apart from this intractable existential capitalist farce, the burning question is how displaced workers will be retrained, incorporated, and reengaged in and around even low-level generative and agentic AI systems.
All-consuming AI
But the flipside – of properly policed and properly empowered AI – is happier democracy, enhanced and energised by its hyped-up brain capacity to make a healthier planet. As an aside, for all the amoral free-market wankers out there, down on meddling EC regulation to put guardrails on AI development, then the message last year from a trade-show stage in Málaga is worth repeating: get this right now, or get it wrong forever, and watch society fail.
So is The Guardian’s view last weekend on AI copyright laws, and Miranda Hyde’s related column in the same paper, on the same day, about big-tech tantrums in glass-houses (“America’s Dumbest Tech Barons”) – as well as her linked-reference to entrepreneur Scott Galloway’s article in Medium that “tech billionaires are the new welfare queens”. But we are getting off track – and perhaps the links to the ‘woke’ press are curious in a business paper.
The original point, here, is to outline the differences of all these modish AI concepts. So let’s continue with that – in pub language, so we are clear. To recap: generative AI is for content creation, product design, and code generation; agentic AI, like an empowered version of gen AI, goes further to make decisions and take actions in a goal-oriented way; and physical AI adds a physical body, like a robot or a vehicle, to an agentic system to interact with the world.
Gen AI creates content based on patterns learned from data, whether generalised content from open-source internet systems, or industry/enterprise content from proprietary libraries. It relies heavily, but not exclusively or necessarily, on large language models (LLMs) to understand and generate human language – such as GPT and Gemini. For domain-specific Industry 4.0 apps, LLMs are typically combined with external knowledge retrieval techniques.
This method, known as retrieval-augmented generation (RAG), improves accuracy. LLMs are used in agentic and physical AI, also – where gen AI is deployed alongside to enable language-based interactions; the same goes with RAG. But gen AI uses other machine learning models, too – such as DALL·E and Stable Diffusion for text-to-image generation, Suno and JukeDeck for text-to-music generation, and Mochi and Open-Sora for text-to-video generation.
Agentic AI, which can exist entirely in software, relies on rule-based systems (in simpler applications), reinforcement learning (to learn to take actions), and planning algorithms (to decide a sequence of actions). Physical AI mixes data from cameras, lasers, and other sensors; it typically uses computer vision models (like YOLO and Mask R-CNN) and deep-learning to identify and navigate objects. AGI, as a concept, combines all of the above, and more.
In summary… actually wait; instead, let’s just quote from everyone’s favourite AI – so we can get on with the dinner; and trust the system and satisfy our SEO engines. And because school kids, today, are being told to ask AI to help with their homework. So here’s the response; all the quotes are from the GPT-4o LLM – developed by OpenAI, which (allegedly) trained on other people’s creations, and co-founded by Sam Altman, who claims to be a victim of the same.
Actually, let’s not. It’s all here. And if you want more on the topic, then check out these articles on recent AI developments by Sean Kinney – who knows a thing or two about a thing or two.
On DeepSeek / AI share-crash:
Bookmarks: DeepSeek (of course), but Jevons’ Paradox really
Dubious DeepSeek debut dings AI infrastructure drive
On NVIDIA at CES / agentic + physical AI:
NVIDIA charts a course from agentic AI to physical AI
NVIDIA takes on physical AI for automotive, industrial and robotics
The three AI scaling laws and what they mean for AI infrastructure
Agentic AI—will 2025 be a breakout year?
On AI fundamentals:
AI 101: The transformational AI opportunity
AI 101: The evolution of AI and understanding AI workflows