From the perspective of big data, the proliferation of internet-of-things (IoT) devices has dramatically changed the way businesses handle data processing. According to BI Intelligence, the velocity of IoT adoption is only set to grow faster. It estimates $70 billion will be spent by global manufacturers on IoT solutions in 2020. It also says 50 per cent of decision-makers in IT, services, utilities and manufacturing have either deployed IoT or will deploy in the next two years.
There is a good reason for this: data is a valuable corporate asset, and its analysis has a dramatic impact on operations. The rise of edge computing means enterprises can retrieve actionable intelligence in near real-time.
But as IoT devices become cheaper, and more numerous, enterprises are confronted with masses of streaming data. They need to be able to process it, fast, if they are to gain timely insights from it. Data loses its value if it is not managed super efficiently.
Awareness of industry best practices will help optimise the process of gleaning insights from streaming IoT data, and yield a return on investment. The open source ecosystem is offering a large number of data-crunching tools which provide a faster, smarter and more capacious way to handle large volumes of data, enabling a range of new and innovative use cases.
Real-time competitiveness
The importance of streaming and analysing data in real-time cannot be understated. In a world where customer experience is built upon factors such as speed, accuracy and efficiency, the need to get actionable insight before data enters the enterprise data lake is a generic one, encountered across a number of industries looking to deploy IoT solutions.
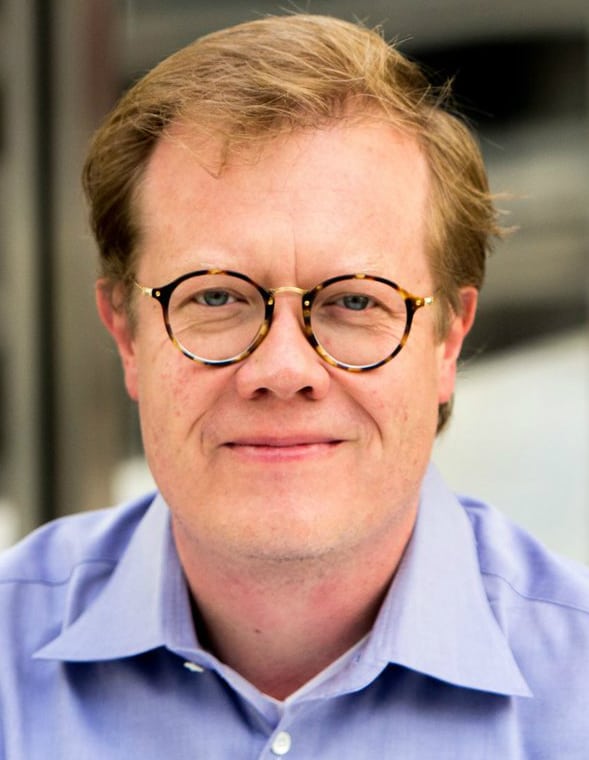
This is, by and large, a result of the fact the conditions in which data is collected in most cases are capricious. Any delay in the data lifecycle between collecting data from customers, analysing it, learning from it, and finally returning it as a bespoke benefit to the customer can greatly diminish its value, even to the point of redundancy.
Also known as ‘data decay,’ this common issue evokes the need for analysis of the data not once it reaches the enterprise data lake (as ‘data at rest’), but whilst it is still contained within the data stream (as ‘data in motion’).
The reasons for countering of data decay become more apparent when looking at some of the major use cases for IoT. For example, in the retail industry – where it is predicted IoT units will grow from 21.6 million to 32.4 million in the period to 2025 – the ability to collect, repackage and deliver timely data insights in order to enhance customer experience and optimise internal operations will be crucial to maintaining a competitive edge.
In the fast-food industry mobile apps are being used not only to allow customers to order from any location, but to ensure food is delivered hot and fresh by using geo-fencing technology to track the customer’s location and prepare their meal just in time for collection.
Timeliness is crucial to executing this added-value service, and demonstrates how, from a business value perspective, narrowing the gap between data ingestion and delivery to the customer increases the value of the data.
Coping with scale
Of course, to enable such use cases, industries must have at their disposal massive-scale data and analytics solutions, capable of processing and fulfilling the entirety of the data lifecycle at scale.
This means finding solutions that address the key challenges of data at scale: volume, velocity, variety, security and governance, all of which are present in the open source community.
Keep in mind that with IoT, the edge is now outside your firewall and very varied. In order to enable real-time data streaming, organisations can rely on readily-available tools such as Apache NiFi, an open-source software project designed to automate the flow of data between software systems through Java, granting the ability to operate within clusters and ensuring security using TLS encryption.
To optimise this process even further, tools such as MiNiFi, a subproject of Apache NiFi, reduce resource consumption by processing data at its point of origin, at the so-called ‘edge’ of the network.
The open source ecosystem is also a breeding ground for deploying machine learning (ML) to accelerate the data lifecycle. Tools such as Apache Spark MLlib feature ML algorithms such as iterative computation, decision trees and clustering to yield better results faster through automation.
In the open-source data landscape, the data lifecycle is imagined across four logical steps:
Ingest – data is transformed and routed visually using Apache NiFi;
Analyse – data is then either sent directly to the datacentre for analysis (either on-prem or in the cloud), or is processed at the edge through MiNiFi agents;
Learn – algorithms and models based on machine learning can be applied to gain critical insights and build business intelligence;
Deploy – deliver instant insights based on perishable data with pattern matching and Complex Event Processing (CEP) from real-time streams.
These open-source data analytics tools, capable of processing data in motion – while also learning from deep analysis of legacy data in a multi-tenant data lake – help to build intelligence for businesses, enhance user experience end-to-end for customers and prevent delays underpinning data decay.
However, the difference now, thanks in part to collaboration, and in part to the number-crunching capacity of modern IT infrastructure, ensures these deliverables at on an enterprise scale.
Where do we go from here?
Every enterprise has a data issue. There will be those which are slightly more or slightly less tech savvy, but the common denominator for these enterprises is the need to address these issues fast.
As the recognition of data as a core business asset emerges in parallel with the rapid uptake of cloud computing solutions, the business strategy of a modern organisation leveraging IoT resources must be built upon these two pillars.
By considering the fact that data is, in fact, a perishable resource, requirement for tools to accelerate the process of data analytics will increase, leading to a rise in the uptake of machine learning algorithms to gain critical insights and build business intelligence in real-time.
This will help businesses to connect with their customers, and maximise the data which customers are willing to provide. No longer will the emphasis be on the connected objects, which mediate the relationship between the enterprise and the customer. Instead, automation of IoT data will cut out the middle-man, and lead to the enterprise interacting with the connected customer.
Scott Gnau is chief technology officer at Hortonworks, a supplier of enterprise-ready open data platforms and modern data applications, based in Santa Clara, California.