By all accounts, 5G is off to a great start in 2021. Adoption has accelerated four times faster than previous LTE technology, resulting in 229 million 5G subscriptions worldwide as of December 2020. Yet even as users embrace the promise of 5G, the increasing complexity and cost of ownership involved in next-generation radio access network (RAN) deployments is vexing network operators.
In today’s mobile network, the RAN represents about 70% of the total infrastructure investment — a considerable amount. Virtualization of the network and its functions were considered to be a key strategy to reduce total cost of ownership for operators. However, this strategy did not target RAN that much. To maximize return on 5G investments, it’s imperative that operators optimize the total cost of ownership (TCO) of the RAN. Virtualized, open radio access network (O-RAN) architecture presents a promising solution to this dilemma, but evolution to O-RAN represents a significant transformation that poses its own challenges as well.
O-RAN introduced a disaggregated and virtualized cloud-native system. It promotes a multi-vendor ecosystem by disaggregating radio with baseband and software from the underlying specialized hardware. It attempts to leverage cloud technologies thanks to economies of scale.
This requires virtualizing the radio network software (or baseband) and opening up and standardizing various interfaces. This architecture promises to improve the overall TCO over time, yet it poses complications for interface validation and system integration. Automation is a top priority for O-RAN network design, but passive automation will not be enough to fully optimize the management of this complex, multi-vendor infrastructure. The future lies in autonomous networks.
Intelligent design
With the capability of leveraging machine learning (ML) and artificial intelligence (AI), autonomous networks can introduce self-healing and automated network operations. Having said that, collecting data from different stack layers to train network behavior is not trivial for RAN, but the introduction of open interfaces in a disaggregated O-RAN network can facilitate that process.
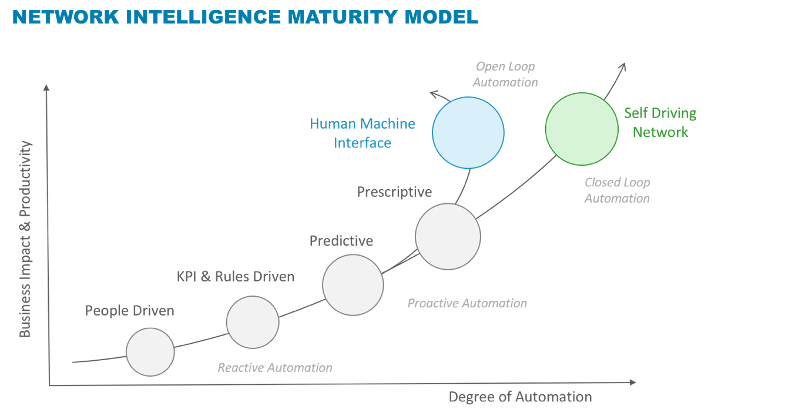
Looking at the evolution of intelligence in network operations, we can see that automation has become the main driver of network intelligence. Going forward, modern proactive automation will become mainstream with autonomous control to provide the predictability for a truly intelligent network.
Intelligent control
In O-RAN architecture, the intelligent automation is facilitated by a RAN Intelligent Controller (RIC). This provides more functions closer to the edge with more interfaces for network managers to leverage. What’s more, this can be implemented on different layers of the RAN and decisions such as optimization at the edge can be made in near real-time.
The RIC enables the development and execution of intelligent applications driven by machine learning (ML), which can implement specific network management operations as cloud-native applications, including mobility management, quality of experience, slicing and admission control.
These applications leverage analytics and data-driven approaches such as advanced ML/AI tools to improve resource management capabilities. This process includes both real-time and non-real-time functionality.
A comprehensive framework is required to accomplish this. The framework needs to:
- Have the capability to collect data from the network
- Train and evaluate different ML and AI models
- Create containerized applications
- Deploy and execute inferring real-time data for application
Moreover, the entire process must rely on continuous learning to improve the accuracy of the trained models. And that is where we must look to next.
Continuous improvement
The O-RAN RIC function is fundamental to the advancement of RAN automation, to improve productivity and performance. And to get there, the next step will require a reference architecture of the RAN Centralized (CU) and Distributed Unit (DU) with sufficient programmability to implement new interfaces. These interfaces can then be bundled into software development kits (SDKs) and offered to RIC application vendors or RAN vendors to enhance the ecosystem. As a result, collected data from the different layers provided by the interfaces can be used to build and package RIC apps, also known as xApps, through easy APIs and consumption models.
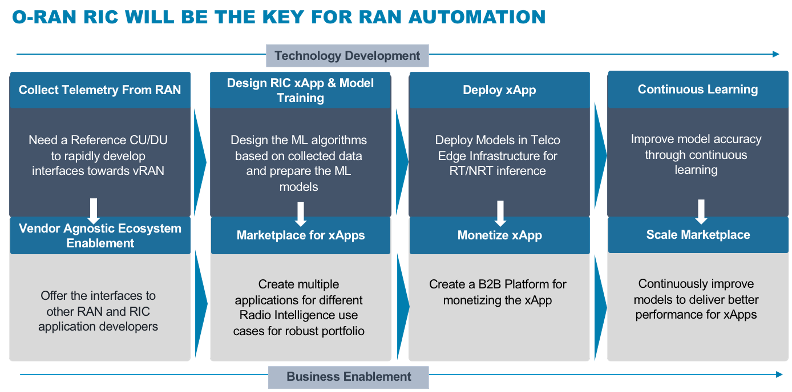
The resulting xApps can then be deployed and monetized — whether for customer benefit, such as improved quality of experience, or to increase productivity and performance of network operations. But the process should not end there.
No machine learning operation should remain static. There needs to be continuous learning and improved performance. Ideally, a marketplace would be established to boost the business model for RIC xApps.
Intelligent RAN could drive the development of new, innovative use cases. For example, a key use case for the RIC function is the ability to optimize quality of experience (QoE). Radio interface data can be collected from multiple user equipment (UE) scenarios through varying traffic profiles, throughput, channel quality indicators (CQI) and hybrid automatic repeat request (HARQ).
Telemetry adaptors can collect data from the RAN layers, and an intelligent app can leverage this to improve radio performance.
Taking this one step further, this same model can be used to accomplish more than improving subscriber experience. Network operations also can be improved through better QoE prediction models, policy models and available bandwidth prediction.
Another use case example is the opportunity to implement and optimize network slicing, an important 5G use case where the separation of various resources and latency parameters is critical. Plus, a similar application could be used to deliver ‘controlled quality of service’ for network applications dependent on network performance.
Self-driving networks
As we look ahead to a brave, new ‘automation-first’ world, the advancement of O-RAN architecture will enable a major transformation. Intelligent, virtualized RAN applications offer network operators several crucial differentiators in terms of new 5G service offerings, network optimization and more efficient operations to reduce TCO. But first, operators will need to foster an environment for ML intelligence to grow and flourish.